Why Analytics Platforms are Failing Your Data Scientists
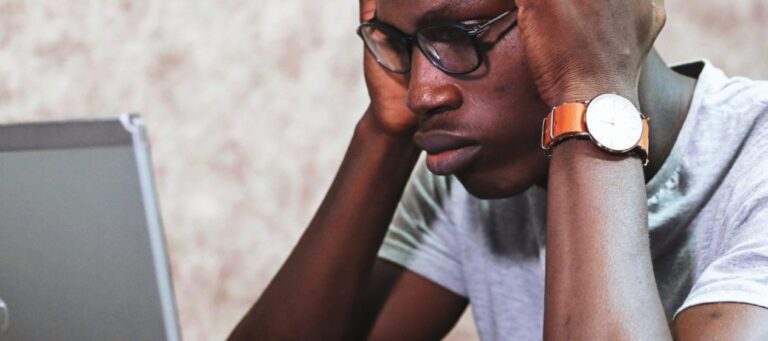
Note: this post was written for and first appeared at Dataconomy: https://dataconomy.com/2019/07/why-analytics-platforms-are-failing-your-data-scientists/
Earlier this year, research firm Market Research Future forecasted the global data analytics market will achieve an annual growth rate of 30 percent through 2023, reaching a market value of almost $78 billion. Yet, according to the Digital Analytics Association, 44 percent of analytics teams spend more than half their time accessing and preparing data rather than doing the actual analysis. That’s a dramatic level of investment for very little return
Is the BI implementation failing because of using the wrong analytics platform? Here are five ways analytics platforms are failing your Data Scientists:
The Person Who Selected Your Analytics Platform Is Not the Person Using It or Benefiting from Its Insights
Despite pure intentions at the beginning of the evaluation process, it’s common to see the functional requirements for analytics platforms weighted disproportionately toward users at the opposite ends of the spectrum. Many analytics platforms cater to the casual user who only lightly consumes information. Other platforms appeal to a narrow band of users who require ultra-sophisticated analytics—the data scientists among the user base. In both cases, your core user base is left with a tool that isn’t a right-sized fit for their everyday needs.
We’ve seen situations where the platform is being evaluated by non-technical users, which can be frustrating for technical staff who require deeper layers of analytic sophistication. We’ve also witnessed situations where the data scientists are making the decision on the tool but don’t necessarily spend a lot of time thinking about business outcomes. Sometimes, both the executive and the data scientist are in the room together, and although the former may in fact be the one making the final decision, the person who will actually be using the tool—the person who will be doing the reports—isn’t asked to weigh in on the decision.
You might say, well, if we want more sophisticated analytics we need to select a platform that prioritizes the data scientist’s needs. Or you might say, to create a culture of analytics, the platform needs to be as easy to use as possible so the greatest number of users want to actually use it. For an analytics implementation to succeed, it needs to be focused squarely on the 80 percent in the middle group of users. The ideal platform finds that middle ground: it provides an accessible User Interface (UI) that the average user can appreciate, but includes sophisticated analytics with simplicity so advanced users can explore more difficult challenges.
Your Analytics Strategy Only Looks Good on Paper
Another common occurrence is a mismatch between the organization’s analytics strategy and the day-to-day analytics and data workflows. This disconnect can arise for several reasons: Oftentimes a consultant or implementation partner assisting with the platform selection lacks a 360-degree-view of the business or comes with preconceived vendor preferences. In other cases, the internally driven vendor selection process is disproportionately weighted to solve a particular use case. Either way, if the analytics tool that’s selected as the centerpiece of the analytics strategy cannot adapt to and accommodate inevitable changes to data or business needs, or if it cannot meaningfully bring users together to collaborate, it will fail.
For example, if you design a prescribed data workflow only to find you cannot connect one data source that’s vital to your analysis and the platform is not able to accommodate it, users may seek a workaround, perhaps an off-the-shelf connector or a different analytics tool altogether. Allowed to continue, you may soon find yourself in a situation where you’re using six different vendors to handle discrete portions of your analytics and data pipeline. What was originally conceived of as a very simple implementation becomes unnecessarily complex.
You can also find yourself in a situation where employees are using their own versions of data or analytics tools that they’re more comfortable using. So, even after you’ve purchased and “implemented” an expensive enterprise analytics platform, you may find that no one’s using it.
A flexible, shared, and governed environment lets you welcome change in the form of new sources and changing infrastructure requirements. An enterprise analytics application needs to eliminate the churn that results from using multiple toolsets. Everyone involved with the decision lifecycle—IT, analysts, data scientists, everyday users—must have the ability to interact with shared, consistent data.
Data Quality is a Constant Headache and Your Data Scientists are Spending More Time Cleansing Data Than Analyzing It
The very reason you opted for an enterprise analytics platform was to harness all your data by bringing it together into a central location. However, while you have access to data, you don’t ever seem to have clean data or data that answers the questions to your most difficult business challenges. And despite the attempt to centralize your data stores, it still resides in different business units or departments. You may have started with an elegant solution on paper, only to find yourself using six different vendors to wrangle one particular data source.
Data Scientists thrive on ready access to data. Without it, they develop workarounds and spend time on less impactful tasks, like data cleansing and normalization. Here’s an all-too-common scenario: a data scientist is asked to prepare an analysis on a data source. If the data isn’t optimized for analysis, they will have to first spend time prepping the data. Then they may prepare their analysis using standalone machine learning (ML) software applications, then output a flat-file for a business analyst to reload into one of several desktop-based BI applications. This results in a perpetual cycle of extracting, importing, analyzing, exporting, re-importing, and re-analyzing data. The whole process is cumbersome and inefficient; meaningful insights derived from AI and ML initiatives remain limited.
Regularizing the process is important for data quality to be high—reproducing (or replicating) data flows is crucial. It’s also important that the mechanism for doing this is intuitive for most users. This is often difficult when you have multiple applications as part of your analytics stack. Bringing more parts of the analytic workflow—prepping data, incorporating ML algorithms, preparing modeling, building visualizations, and assembling dashboards and reports—into a single application makes it easier to recreate (and automate) data workflows.
You’re So Focused on Optimizing Your Machine Learning Workflow That You’re Missing the Big Picture
The wrong analytics tool—or completely standalone ML applications—can isolate your data scientists from the everyday practice of analytics. If the tool doesn’t provide an environment where advanced users can collaborate with typical platform users, the whole process fragments further. So, now not only are there data silos, there are analytics islands—distinct user types are performing their own analyses with their own applications.
Data Scientists thrive when they’re building out algorithms, setting model parameters, and testing results. With the wrong tool, most of the actual work they do is far more mundane: data cleansing, maintaining data stores, figuring out how to meaningfully share results back out to business users. An analytics platform should ideally make the not-so-fun part much easier so the data scientists can put their models, algorithms, and reports into a production environment much more quickly.
The Ad-Hoc Nature of the Business Strains Your Advanced Analytics Users
You most likely established AI and ML initiatives because you recognized that to up-level analytics capabilities you had to commit to hiring Data Scientists, invest in Big Data infrastructure, and choose analytics technologies that could bring advanced insights into typical business scenarios.
What often happens though is the organization’s general enthusiasm to embrace analytics, combined with the ad hoc nature of the business, quickly overwhelms your data science resources. For example, say the VP of Marketing comes to the data science team to ask which targeted social advertising campaigns and audiences are signaling the highest intent to purchase based on past behavior. Then the VP of Sales asks which products and markets they should prioritize based on current sales figures. In isolation, each request is reasonable and valuable. However, if your data science teams are doing all that outside of your normal data flow (with standalone tools), the process from an employee resource perspective becomes inefficient and becomes disconnected from the broader organization.
Without a rigorous process for managing these advanced projects, your data scientists are quickly stretched thin: they duplicate time-consuming work, and they don’t provide value to the organization from a broader strategic perspective. If you’re using a platform that brings all of this together in a single environment, you can put models into production much more quickly and readily. Plus, the results are much more integrated and available.
What to Do Instead
In this article, we’ve articulated all the key ways the wrong analytics platform can fail your Data Scientists. We’ve found that the recipe for success includes end-to-end analytics platforms that target the broadest set of users. The key is to create an analytics environment that provides specific toolsets and functionality that are valuable to any participant in the decision lifecycle, end-users and data scientists alike. This increases value not only at the department level but across the enterprise. While user adoption increases, IT and analytics leaders maintain vital visibility into data consumption. That way, analytics can finally start to deliver actionable recommendations for all business needs across the enterprise.